AUXAL 4 結構分析軟體
- AUXAL 4 結構分析軟體
-
類別統計分析軟體
-
介紹縱向發展研究的全面資料分析,現在利用SSI的AUXAL程式在您桌面就可使用。 BTT模型或JPA2模型之結構分析 用於數據分析的NCSS軟件配有集成文檔,免費培訓視頻以及博士統計人員團隊提供的完整電話和電子郵件支持。 殘值或Kernel Smoothing的傅立葉變換之非結構分析
AUXAL 4 Structure Analysis Software
A new version of the AUXAL program is immediately available for purchase.
Auxal4 uses the same empirical Bayes estimation procedure as
AUXAL3, but has new features and improvements that extend the
functionality of the program and make it easier to use. The accuracy of the estimated population covariance matrix of the model parameters has been improved. Although archival of data from the Fels longitudinal study continues as the source of the default prior distribution means and covariance matrices for the several models, the more accurately estimated covariance matrices provide
the priors of Auxal4. When the user wishes to replace of the
priors in the current job by those based on other longitudinal
data, the steps involved have been simplified (see MEAN and COVARIANCE commands below).
The evaluated heights of the structural average curve that appear at the end of the summary output listing now include standard
errors of height at any given age. They facilitate statistical analysis of group comparisons of structural average curves. The standard errors are computed from the estimated population covariance matrix of the parameters and the derivatives of the structural average curve with respect to the parameters at any given age point (see Rao, C. R. (2002). Linear Statistical Analysis and Its Applications, 2nd edition, paperback, New York: Wiley, pp.386-389). If the
cases in the current analysis are drawn from the same population as the assumed prior distribution, the default covariance matrix
of the prior is used in these computations. If the size of the current sample is large enough to justify large sample assumptions,
the population covariance matrix estimated in the current job
may be used in place of the default (see TECHNICAL command
below).
Facilities have been added for cross-sectional analysis of mixed longitudinal data. They include standard errors for average height in given age intervals, and a provision for evaluating so-called plausible values of height at any given age that permit conventional multivariate analysis of group differences in mixed longitudinal data. The standard errors are exact when each case is represented by only one observed height in each interval. Otherwise it is conservative: additional observations within the interval that information, but it is difficult to evaluate the reduction in the standard error because of the observations are correlated.
Changed commands, options, and keywords
MEAN and COVARIANCE commands
New option: IMPORT
These commands allow the user to replace the prior mean and covariance matrix with others more relevant to the current analysis. If new versions of the population mean and covariance matrix have been estimated by the program and saved using the MEAN keyword of the SAVE command, the appearance of the new option in these commands automatically extracts the mean and/or covariance matrix from an existing file created by the SAVE command. In the absence of the IMPORT option, the mean or covariance matrix will be read from existing files containing the parameter values in the standard order described in the AUXAL manual.
ERROR command
Setting larger error standard deviations (SD) for purposes of suppressing failures of the maximum posterior eye estimation procedure from converging is no longer required globally. It is now applied only to those cases that do not converge in the iterative estimation of the model parameters for the case. When this occurs, the program automatically attempts re-estimation up to five times with increasing values of the error SD. If convergence is then obtained, the last value of the SD appears in the case processing list. These adjustments typically reduce the number of failed convergences. (Failures of convergence are also fewer when autocorrelation of the residuals across ages is neglected by invoking and the TECHNICAL option UNCOR.
The ERROR command is no longer needed, but is still operative.
TECHNICAL command
New option: SAMPLE
If this option is present, the program will base the standard errors of the structural average curve at any given age on the population covariance matrix of the model parameters estimated in the current job. Otherwise, the default prior distribution is the source of the covariance matrix in the computations.
New keyword: PLSEVAL = t
If the EVALUATE key word of the PROCEDURE command is present, and the evaluated heights of the cases at successive ages are saved using the EVAL keyword of the SAVE command, the evaluated heights are converted into plausible values on the assumption that the measurement error distribution at each age has mean zero and standard deviation equal to the square root of the error variance for the individual case. A random deviant from this distribution is added to each of the evaluated heights. Group differences of cross-sectional average growth can be analyzed by multivariate analysis of variance using these values as data. Their sampling variance includes the effects of sampling the cases as well as that of those of measurement error and the equation error (see also SAVE command).
The quantity t is the seed of the random number generator—any integer greater than 1 and less than 2147483647.
New keyword: ONLY = u
This keyword allows the program to compute the standard errors of a structural average curves directly from user-supplied values of the parameters means (possibly those from analyses by other investigators). The parameters must be input by use of the MEAN command in the standard order for the model in question. The quantity u is the number of cases in the sample from which the putative mean was obtained. The program must be then executed in a dummy job of least a few cases. The plot of the curve follows as usual. The default prior covariance matrix is used in these calculations.
SAVE command
Keyword: COVARIANCE
This keyword was not implemented in AUXAL3.
It is now operative.
Option: HGTROW
If case heights or plausible value heights are evaluated, this option lists the output in rows of space-delimited values; otherwise, the values will be listed in a single column.
Adjusting priors for different populations
If measurements of a large sample of N cases from a suitable longitudinal growth study are available, the population mean covariance matrix of the model parameters can be estimated from the MAP estimate and posterior covariance matrix for each case.
Because the population mean and covariance matrix are required in the prior distribution for estimating the parameter means and covariances, a “boot strap” procedure is required in their use. Initially, one starts with the existing AUXAL priors for the BTT, JPA2 or Jenss-Bayley models. These priors are based on USA data. Provided the number of well-spaced data points per case exceeds, say, 20, a pass through the cases with this provisional prior will give a good approximation to the population quantities. The revised prior can be saved to an external file via the SAVE command
>SAVE means=’priors.par’;
The file priors.par contains the estimated population mean and population covariance matrix of the model parameters.
A second or third pass, each time substituting the resulting provisional prior will yield a sufficiently accurate estimation of the population mean and covariance matrix for practical use in MAP estimate of the model parameters. The prior obtained from the previous run is read into the program via the commands
>MEAN MALE FILE = ‘male.mea’;
>COVARIANCE MALE FILE = ‘male.cov’;
See examples exampl10.axl and exampl11.axl.
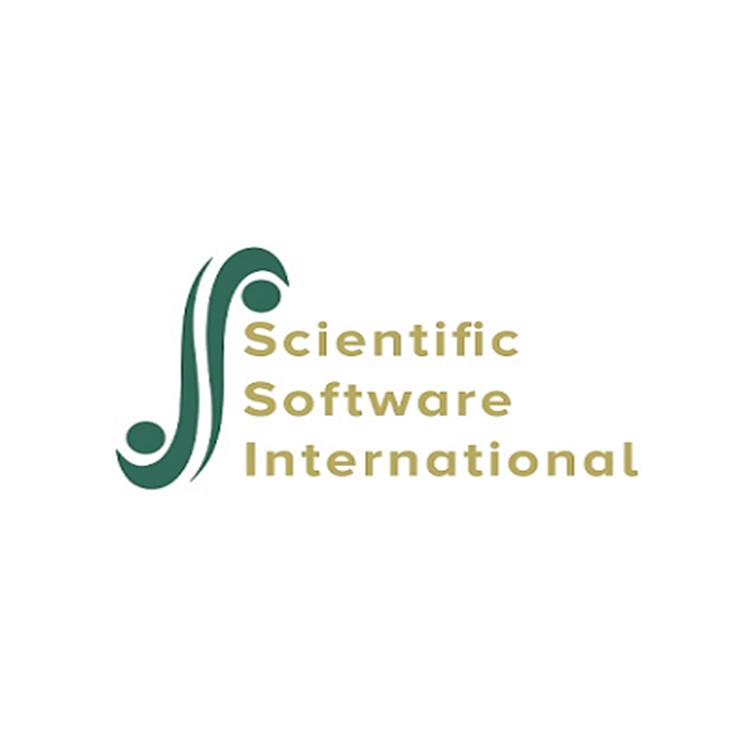
系統需求
Microsoft Windows XP, 2000, Vista, Windows 7, 8 and 10
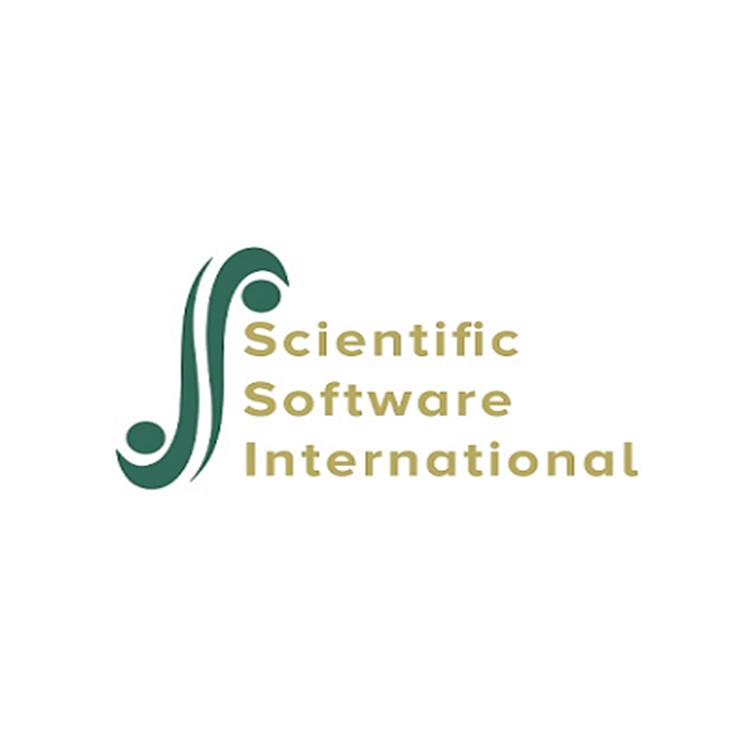
AUXAL 4 結構分析軟體
Auxal4 使用與 AUXAL3 相同的經驗貝葉斯估計過程,但具有擴展程序功能並使其更易於使用的新特性和改進。模型參數估計總體協方差矩陣的準確性得到了提高。儘管來自 Fels 縱向研究的數據存檔繼續作為默認先驗分佈均值和幾個模型的協方差矩陣的來源,但更準確估計的協方差矩陣提供了 Auxal4 的先驗。當用戶希望將當前作業中的先驗替換為基於其他縱向數據的先驗時,所涉及的步驟已被簡化(請參閱下面的 MEAN 和 COVARIANCE 命令)。
出現在匯總輸出列表末尾的結構平均曲線的評估高度現在包括任何給定年齡的身高標準誤差。它們有助於對結構平均曲線的組比較進行統計分析。標準誤差是根據參數的估計總體協方差矩陣和結構平均曲線相對於任何給定年齡點參數的導數計算得出的(參見 Rao, CR (2002)。線性統計分析及其應用,第 2 版,平裝本,紐約:Wiley,第 386-389 頁)。如果當前分析中的個案來自與假定先驗分佈相同的總體,則在這些計算中使用先驗的默認協方差矩陣。如果當前樣本的大小足以證明大樣本假設的合理性,
添加了用於混合縱向數據的橫截面分析的設施。它們包括給定年齡區間平均身高的標準誤差,以及評估任何給定年齡所謂的合理身高值的規定,允許對混合縱向數據中的組差異進行傳統的多變量分析。當每個案例僅由每個間隔中的一個觀察高度表示時,標準誤差是精確的。否則它是保守的:在該信息的區間內附加觀測值,但由於觀測值是相關的,因此很難評估標準誤差的減少。
更改了命令、選項和關鍵字
MEAN 和 COVARIANCE 命令
新選項:導入
這些命令允許用戶用與當前分析更相關的其他命令替換先驗均值和協方差矩陣。如果程序已估計新版本的總體均值和協方差矩陣並使用 SAVE 命令的 MEAN 關鍵字進行保存,則這些命令中出現的新選項會自動從創建的現有文件中提取均值和/或協方差矩陣通過 SAVE 命令。在沒有 IMPORT 選項的情況下,將從包含 AUXAL 手冊中描述的標準順序的參數值的現有文件中讀取均值或協方差矩陣。
錯誤指令
全局不再需要為了抑制最大後眼估計過程的收斂失敗而設置更大的誤差標準偏差 (SD)。它現在只應用於那些在模型參數的迭代估計中不收斂的情況。發生這種情況時,程序會隨著誤差 SD 值的增加自動嘗試重新估計最多五次。如果隨後獲得收斂,則 SD 的最後一個值出現在案例處理列表中。這些調整通常會減少收斂失敗的次數。(當通過調用和 TECHNICAL 選項 UNCOR 忽略跨年齡殘差的自相關時,收斂失敗也更少。
ERROR 命令不再需要,但仍然有效。
技術指令
新選項:SAMPLE
如果存在此選項,程序將根據當前工作中估計的模型參數的總體協方差矩陣,將任何給定年齡的結構平均曲線的標準誤差作為基礎。否則,默認的先驗分佈是計算中協方差矩陣的來源。
新關鍵字:PLSEVAL = t
如果存在 PROCEDURE 命令的 EVALUATE 關鍵字,並且使用 SAVE 命令的 EVAL 關鍵字保存連續年齡案例的評估高度,則評估的高度將轉換為合理值,假設測量誤差分佈在每個年齡的均值為零,標準差等於個別情況的誤差方差的平方根。這種分佈的隨機偏差被添加到每個評估的高度。可以使用這些值作為數據通過多變量方差分析來分析橫截面平均增長的組差異。它們的抽樣方差包括抽樣的影響以及測量誤差和方程誤差的影響(另見 SAVE 命令)。
數量 t 是隨機數生成器的種子——任何大於 1 且小於 2147483647 的整數。
新關鍵字:ONLY = u
此關鍵字允許程序直接根據用戶提供的參數均值(可能來自其他調查人員的分析)計算結構平均曲線的標準誤差。必須使用 MEAN 命令按相關模型的標準順序輸入參數。數量 u 是從中獲得推定平均值的樣本中的案例數。然後必須在至少少數情況下的虛擬作業中執行該程序。曲線圖照常如下。在這些計算中使用默認的先驗協方差矩陣。
保存命令
關鍵字:協方差
此關鍵字未在 AUXAL3 中實現。它現在可以運行了。
選項:HGTOW
如果評估大小寫高度或合理值高度,則此選項以空格分隔值的行列出輸出;否則,這些值將列在單個列中。
調整不同人群的先驗
如果可以從合適的縱向增長研究中測量大量N 個案例的樣本,則模型參數的總體平均協方差矩陣可以從每個案例的 MAP 估計和後驗協方差矩陣中估計出來。
由於在先驗分佈中需要總體均值和協方差矩陣來估計參數均值和協方差,因此在使用它們時需要“引導程序”程序。最初,從 BTT、JPA2 或 Jenss-Bayley 模型的現有 AUXAL 先驗開始。這些先驗是基於美國的數據。如果每個案例中間隔良好的數據點數量超過 20 個,則通過具有此臨時先驗的案例將很好地近似總體數量。修改後的先驗可以通過 SAVE 命令保存到外部文件中
>SAVE means=’priors.par’;
文件priors.par包含模型參數的估計總體均值和總體協方差矩陣。
第二遍或第三遍,每次替換產生的臨時先驗將產生對總體均值和協方差矩陣的足夠準確的估計,以用於模型參數的 MAP 估計中的實際使用。從上次運行中獲得的先驗通過命令讀入程序
>MEAN MALE FILE = ‘male.mea’;
>COVARIANCE MALE FILE = ‘male.cov’;
請參見示例example10.axl和example11.axl。
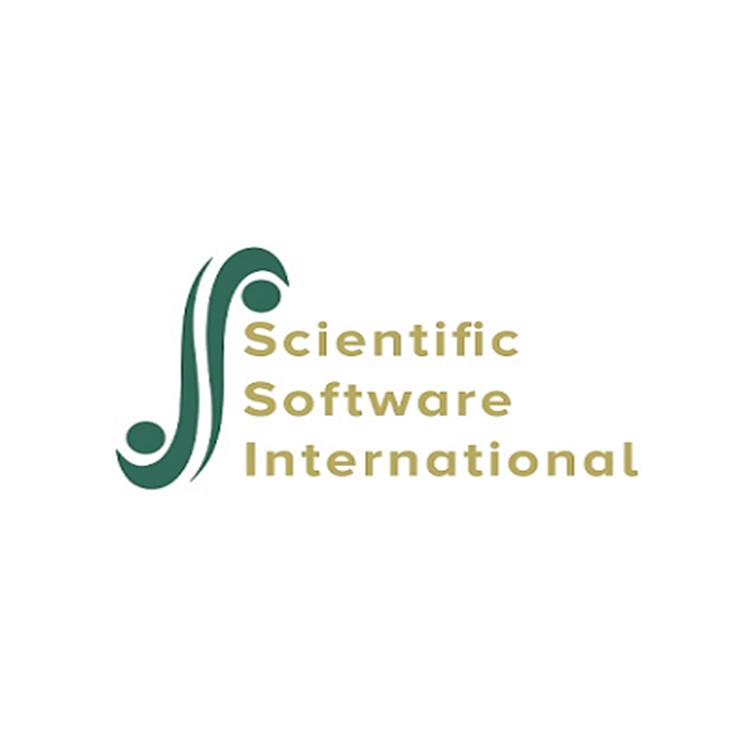
UNISTAT 10.11 統計分析套裝軟體
UNISTAT是視窗型統計分析軟體,運行於Windows平臺之上,其統計分析功能齊全、多種運行模式、介面友好、可操作性強、資料輸人輸出靈活。
AUXAL 4 結構分析軟體
縱向發展研究的全面資料分析,現在利用SSI的AUXAL程式在您桌面就可使用。 BTT模型或JPA2模型之結構分析 用於數據分析的NCSS軟件配有集成文檔,免費培訓視頻以及博士統計人員團隊提供的完整電話和電子郵件支持。 殘值或Kernel Smoothing的傅立葉變換之非結構分析
LISREL 結構方程式模型軟體
LISREL是一款專業且功能強大的統計分析軟體,主要用於結構方程模型(SEM)建模。它允許研究人員建立複雜的統計模型,用於檢驗理論和研究不同變數之間的關係。透過LISREL,用戶可以使用統計模型來檢驗假設,了解變數之間的相互關係,以及這些關係如何影響觀察到的數據,並且執行多組群體差異比較。它支援不同類型的變數,包括觀察變數和潛在變數,並且能夠處理複雜的數據結構。LISREL還提供了豐富的統計分析功能,包括驗證性因素分析(CFA)、路徑分析和因果關係等。這些功能使得用戶能夠對數據進行全面的分析,從而深入理解研究問題和現象。此外,LISREL還具有強大的模型擬合和評估功能,用戶可以通過適度性指標分析來評估模型的有效性和適合度,這有助於確保建立的模型能夠合理地解釋觀察到的數據,提高研究的可靠性和信度。